Nowadays, businesses are leaning a little more towards, data analytics for data modeling and engaging valuable customers. With a relatively clear strategy for utilizing historical data patterns, even the non-technical business owners integrate it within their infrastructure. Most of them are unaware of the impact that predictive analytics can make to drive better future outcomes for their businesses.
Back in 2015, Harvard University and Yelp in partnership with popular data science platform, DrivenData designed predictive analytics solutions for health inspection in restaurants. Although, predicting future trends takes a lot of your time and various strategies that may or may not land on the targeted spot to reach the predict future outcomes.
Read along to know more about predictive analytics and applying analytics techniques to increase business efficiency.
What Is Predictive Analytics?
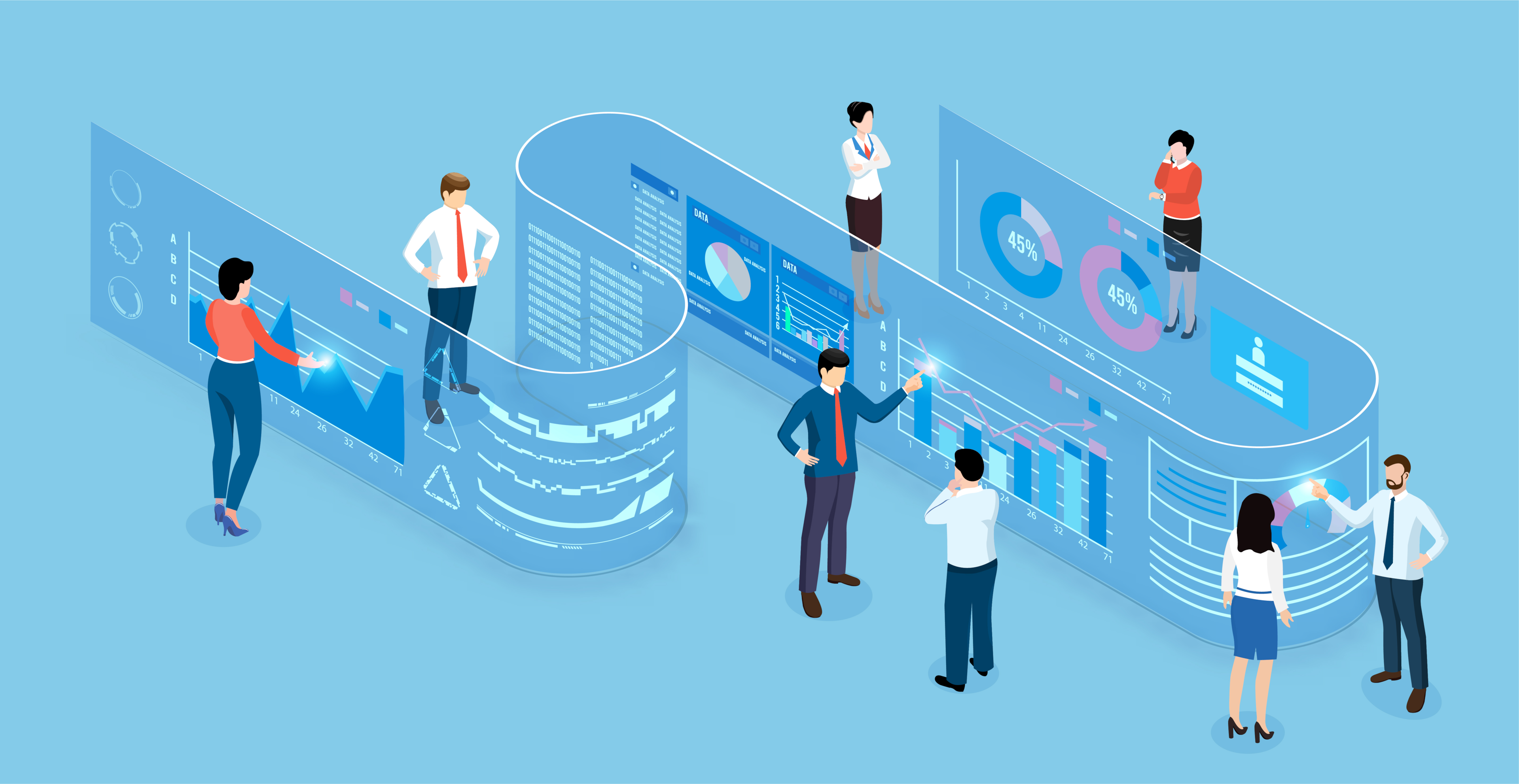
Predictive analytics reflect the use of historical data relevant to train machines, to identify risks and determine credit scores within your business to predict future outcomes. Predictive analytics enables organizations to get fresh insights from their data and resolve business issues, such as choosing which items to advertise to particular client categories and on which platforms. Predictive models mathematically entail estimating a mapping function (f) from input variables (X) to output variables (Y).
The most common use cases include optimizing marketing campaigns based on historical customer data, detecting fraud by analyzing patterns of criminal behavior, and forecasting inventory or setting prices. For example, airlines use predictive analytics to set ticket prices by forecasting seat availability and demand.
In fact, predictive analytics is an increasingly important decision-making tool. The ability to merge data from multiple sources and improve prediction accuracy empowers businesses to gain insights from their data. The global market for predictive analytics is set to attain a $10.95 billion valuation by 2022, up from $3.49 billion in 2016, according to a report issued by Zion Market Research.
Aside from helping businesses to acquire and retain customers, predictive analytics also helps businesses minimize risk and detect criminal behavior.
Big Data and Predictive Analytics Techniques
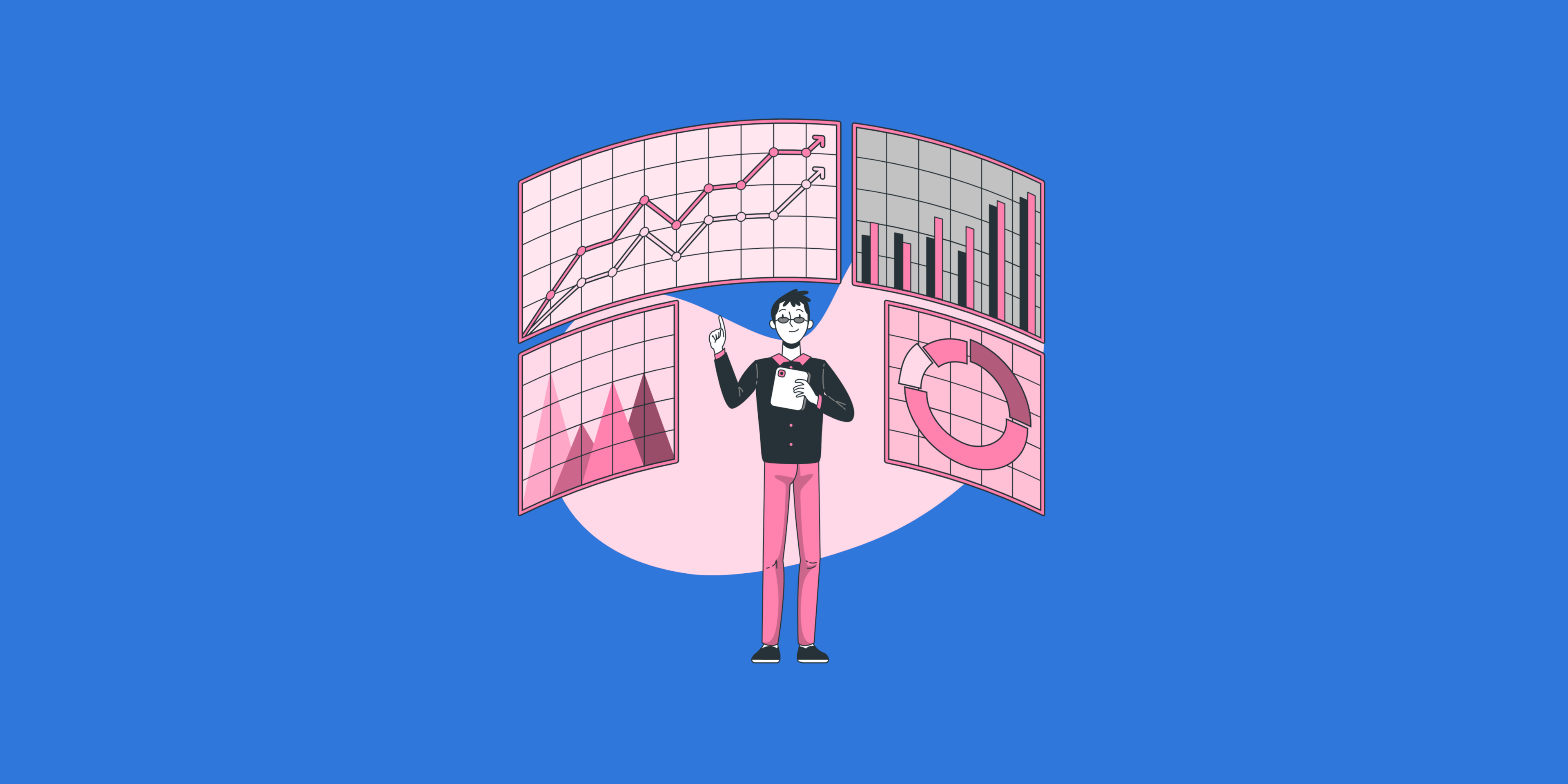
Since that predictive analysis depends on data, wouldn't having a lot of data available indicate accurate predictive analysis? Unexpectedly, it wouldn't. Few businesses are able to interpret all the data they are gathering, despite the fact that many exhibit interest in predictive analytics techniques.
In fact, a lot of businesses analyze data using descriptive analytics. Predictive analytics examines past data, but it also seeks to predict future patterns and trends that may emerge in the future. Then, it bases judgements on those predictions.
Thus, every chunk of big data falls within the category of predictive analysis. In light of this, let's examine some specific predictive analytics techniques that serve as the foundation for precise prediction in addition to data analysis.
Top 10 Predictive Analytics Techniques
Following are the ten best predictive analytics techniques that ensure boost within your business performance:
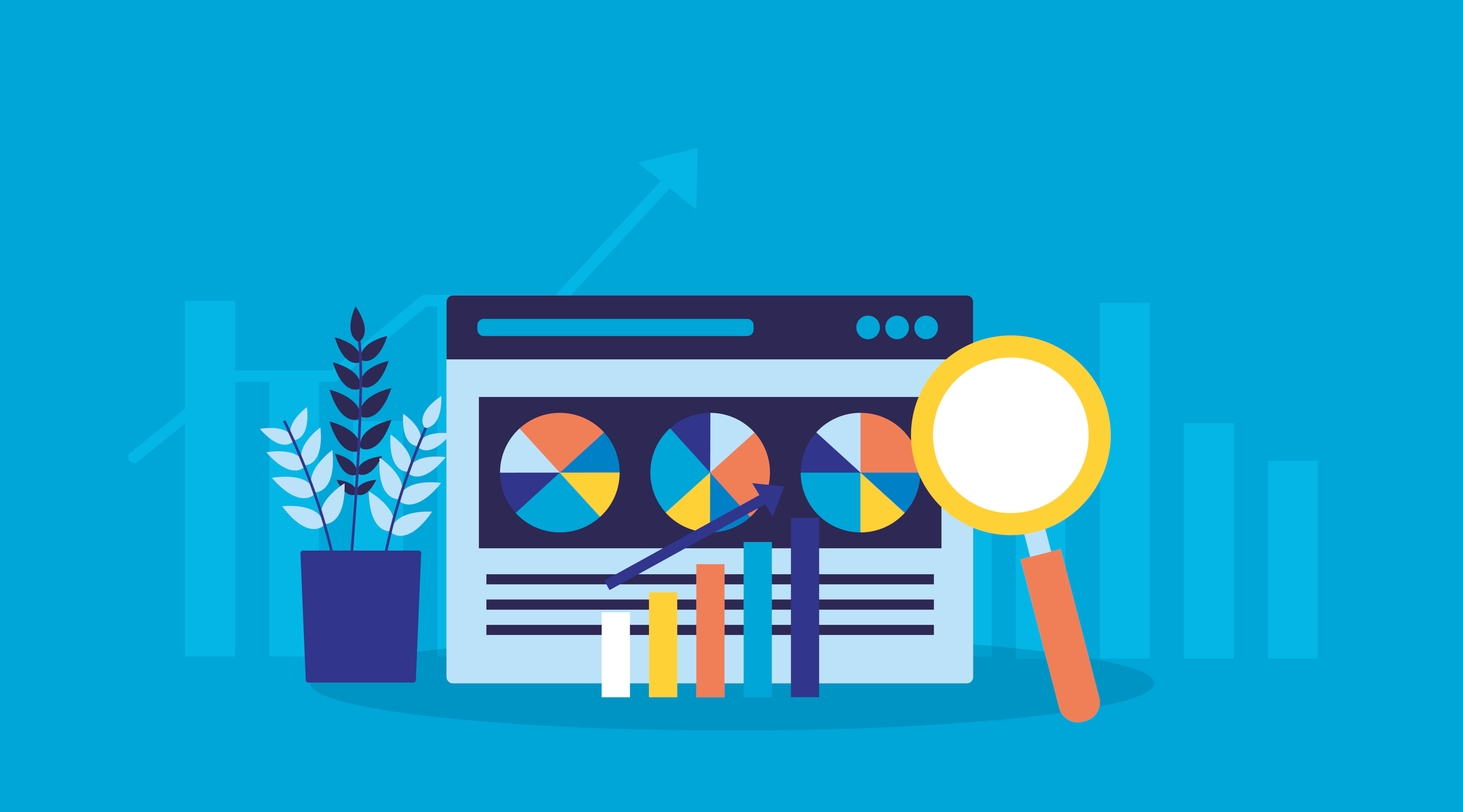
Data mining
To find anomalies, trends, and correlations in huge datasets, data mining is a technique that combines statistics with machine learning. Businesses can use this method to transform raw data into business intelligence, which includes current data insights and forecasts that help with decision-making. Data mining is the process of sifting through repetitious, noisy, unstructured data to find patterns that reveal insightful information.
Exploratory data analysis is a form of data mining methodology that entails reviewing datasets to highlight their key features, frequently using visual techniques. EDA focuses on probing the facts in an objective way without any expectations; it does not entail hypotheses or the deliberate search for a solution. The goal of traditional data mining, in contrast, is to use the data to develop solutions or to address a specific business problem.
Classification
A prediction approach called classification involves estimating the likelihood that a given item falls into a particular category. A multi-class classification problem is one that has more than two classes, as opposed to a binary classification problem, which only has two classes. Classification models produce a continuous number, usually referred to as confidence, that reflects the likelihood that an observation falls into a specific class.
The class with the highest probability can be chosen to represent a predicted probability as a class label. Spam filters, which categorize incoming emails as "spam" or "not spam" based on predetermined criteria, and fraud detection algorithms, which highlight suspicious transactions, are the most prevalent examples of categorization in a business use case.
Regression Model
Regression analysis is a type of predictive modelling technique that looks at how an independent variable and a dependent variable are related, meaning it predicts how the attacker impacts the target. Forecasting, time series modelling, and determining the link between the variables are all done using this method. For instance, the association between careless driving and the number of accidents a driver causes on the road can be analyzed through regression predictive algorithms.
Modelling and analyzing data make predictive analytics important due to its flexibility. Here, we try to minimize the disparities between the data points' distances from the curve or line by fitting a curve or line to them. In the sections that follow, I'll go into further depth about this.
Regression analysis has a variety of advantages, including:
Highlighting the important connections between the dependent and independent variables.
Showing how strongly an independent variable has an impact on a dependent variable.
We may compare the effects of variables measured on various scales, such as the impact of price adjustments and the volume of promotional activities, using this method as well. These benefits help market researchers, data analysts, and data scientists choose the best set of variables to use when building new products. These advantages aid market researchers, data analysts, and data scientists in identifying and selecting the ideal set of variables to use for predictive models.
Clustering
One of the most often used data mining techniques is clustering, which divides a huge dataset into smaller chunks by categorizing objects based on their similarity into groups. When consumers are grouped together based on shared purchasing patterns or lifetime value, for instance, customer segments are created, allowing the company to scale up targeted marketing campaigns.
Hard clustering entails categorization of data points directly. Instead of actually assigning a data point to a cluster, soft clustering gives it a likelihood that it fits in one or more clusters. One of the most widely used autonomous machine learning algorithms is K means clustering. Using a target number k, this strategy searches a dataset for a predetermined number of clusters.
Predictive modeling
Predictive modeling is a statistical modeling technique in which probability and data mining are used to predict future events. These predictions are then used to inform future actions or decisions, such as determining how much fresh produce a restaurant should stock on a federal holiday or how many calls a customer support agent should be expected to field in one day.
Artificial intelligence and machine learning
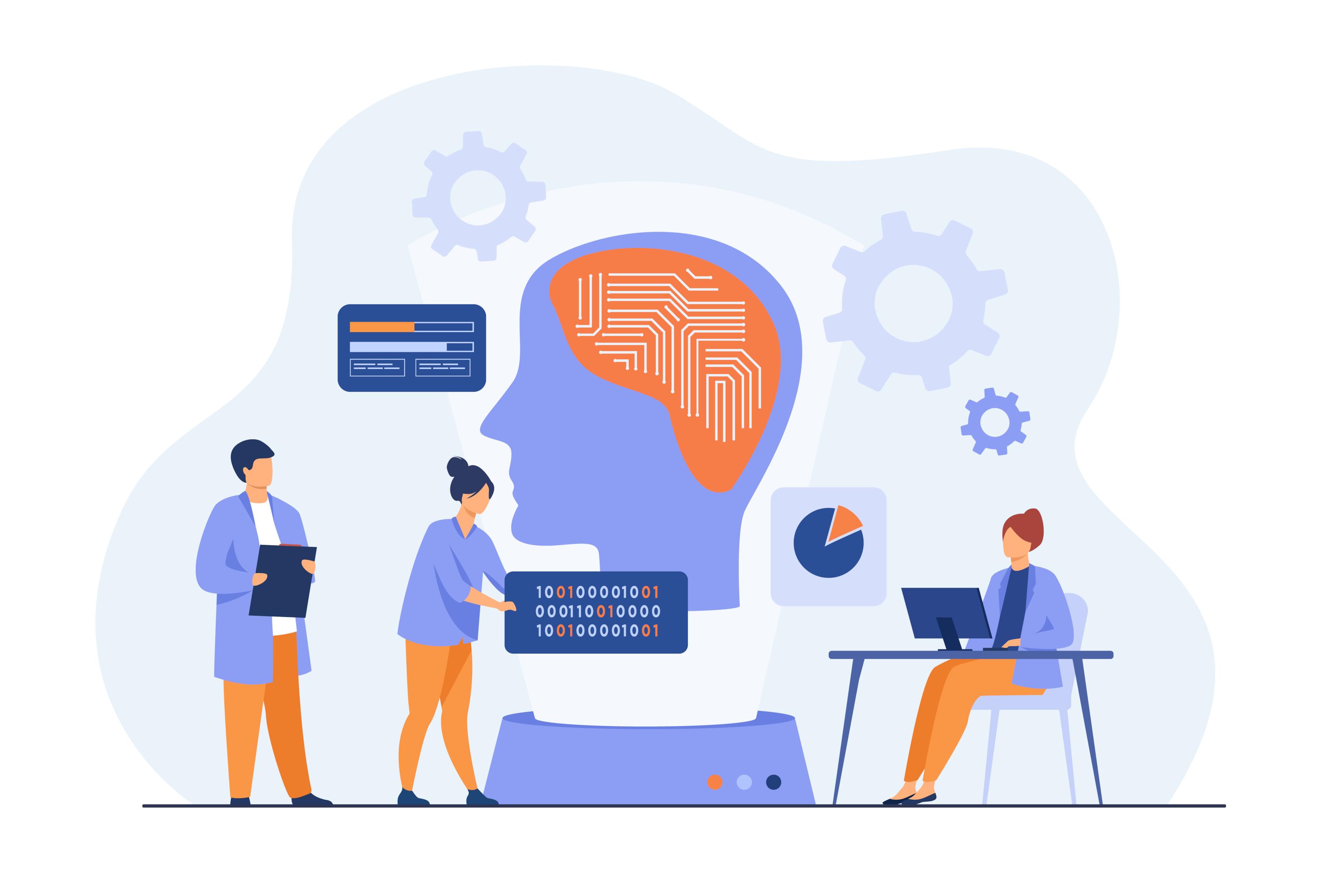
Machine learning is a type of computational learning that examines data and builds models that fit the data in the context of predictive modelling. For instance, the algorithm will forecast income as a function of GPA if historical data indicates that students with higher GPAs often earn greater earnings. Sadly, these machine learning models are effectively "black boxes," created by machine learning without any explicit programming by humans. They are created directly from the data.
As a result, the caliber of the training data is crucial to how well machine learning algorithms perform. The accuracy of the model's predictions is weakened by data that is skewed, out of date, or insufficiently representative of the target population.
Decision trees
A decision tree is a well-liked tool for displaying analytical models and a supervised learning procedure. Using a succession of "if...then" statements (sometimes referred to as indicators) arranged in the shape of a flow diagram, decision trees categorize inputs into two or more categories. To forecast continuous quantitative data, like a person's income, a regression tree is utilized.
A classification tree is used in qualitative predictions, such as a tree that forecasts a diagnosis based on a patient's symptoms. By learning straightforward decision rules derived from training data, a decision tree can be used to build a training model that can determine the category or value of an input variable.
Neural networks
Neural networks, which are frequently employed to solve data classification issues, were biologically inspired by the human brain. The majority of neural networks employ mathematical equations that pair each input with a corresponding output to activate the neurons.
Create a web of input nodes (where you insert the data), output nodes (which display the findings after the data has travelled through the networking), and a hidden layer between each of these nodes to create a neural network. Because the hidden layer "learns" like a human would by remembering previous connections in data and applying this info in the algorithm, the network is better than conventional predicting tools.
However, this hidden layer in neural networks represents a ‘black box,’ meaning that even data scientists cannot necessarily understand how predictive analytics produces its results—only the inputs and outputs can be observed directly.
Logistic regression
One of the most important tools for predictive modelling is logistic regression modelling. Finding correlations between inputs and outputs in the form of a linear expression that expresses the strength of the link as a mathematical formula is the fundamental goal of regression approaches.
With the addition of a constant, the formula represents the outputs as a function of the inputs. In order to forecast a variable's future numerical value, this linear connection is then applied.
A regression model, for instance, can demonstrate the relationship between home prices and interest rates and then utilize the linear expression to forecast future home prices given an interest rate of 'X. The variables used to predict the value of the dependent variable are known as independent variables, whilst the variable being predicted is referred to as the dependent variable.
Regression models consist of two parts: multiple linear regression and simple linear regression. Simple regression techniques include one dependent variable and one independent variable, meanwhile, multiple regression techniques involve one dependent variable and multiple independent variables.
Time series analysis
Another predictive analytics technique is the time series predictive model for analyzing time-series data. Time series models forecast future values using values that have already been observed.
A time series is a collection of data items that happen repeatedly over time, such as variations in the median household income or changes in the price of a stock. The expected values in this scenario follow a temporal continuum.
These predictive analytics models are helpful for making judgements that entail an element of uncertainty over time or when projecting behavior or metrics over time.
Best Predictive Analytics Tools
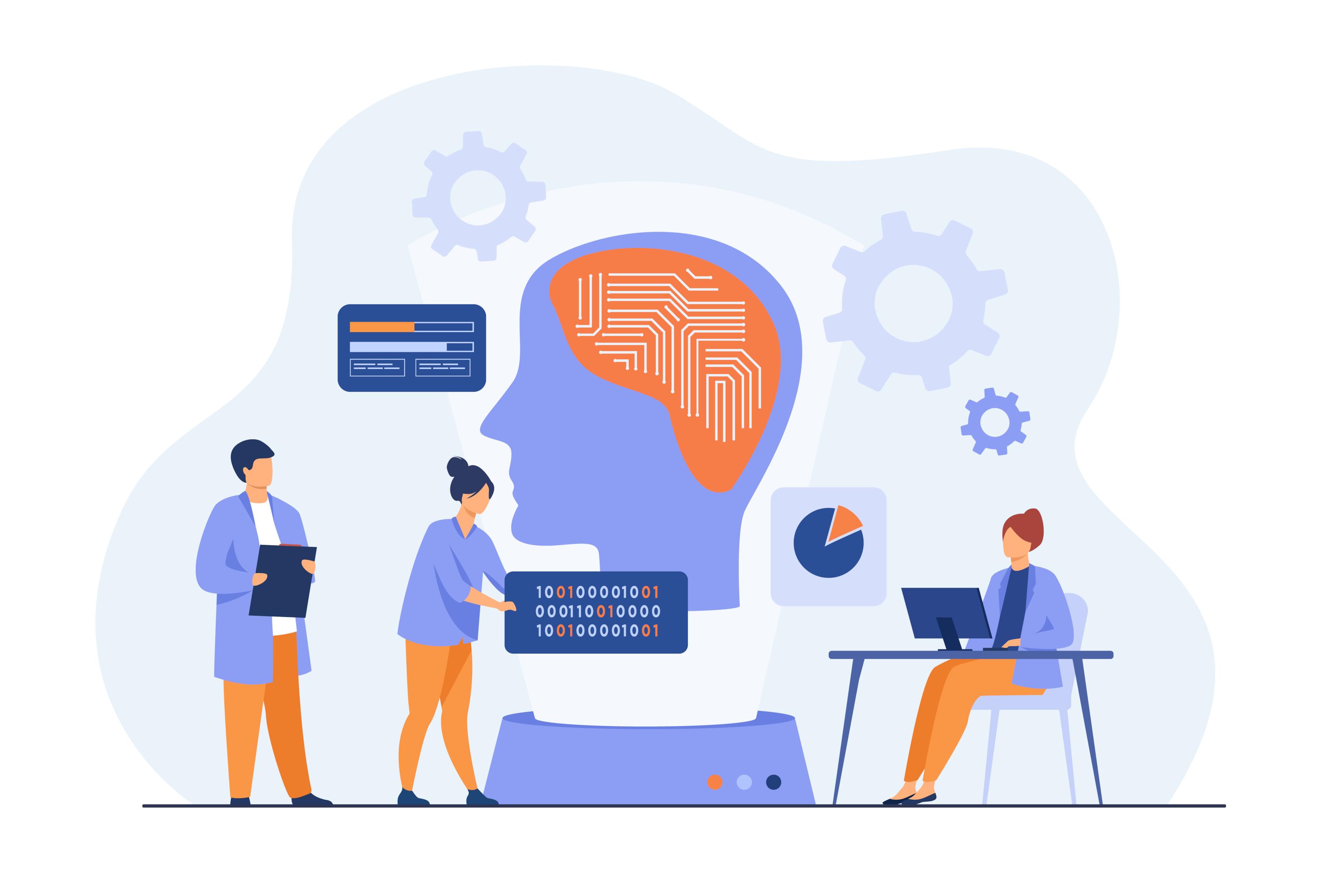
Among some of the best predictive analytics tools, a few of them include:
- Tableau
- Qlik Sense
- IBM Watson Studio
- RapidMiner
- Adobe Analytics
- SAP Predictive Analytics
Benefits of Predictive Analytics Techniques
Although there are many different benefits of implementing predictive analytics within your businesses, some of them are listed as follows:
Personalize the customer experience.
Mitigate risk and fraud.
Proactively address problems.
Reduce the time and cost of forecasting business outcomes.
Gain a competitive advantage.