Amazon Web Services is the name that has completely revolutionized the computing world! How? Amazon Web Services or AWS is a cloud service provided by Amazon in which you can find different building blocks as services to develop and implement any type of cloud application. The various AWS services are designed to work in unison with each other to develop highly sophisticated and scalable applications.
Why is Data Analytics Important?
Data analytics helps companies gain more visibility and a deeper understanding of their processes and services. It gives them detailed insights into the customer experience and customer problems. By shifting the paradigm beyond data to connect insights with action, companies can create personalized customer experiences, build related digital products, optimize operations, and increase employee productivity.
How Does Big Data Analytics Work?
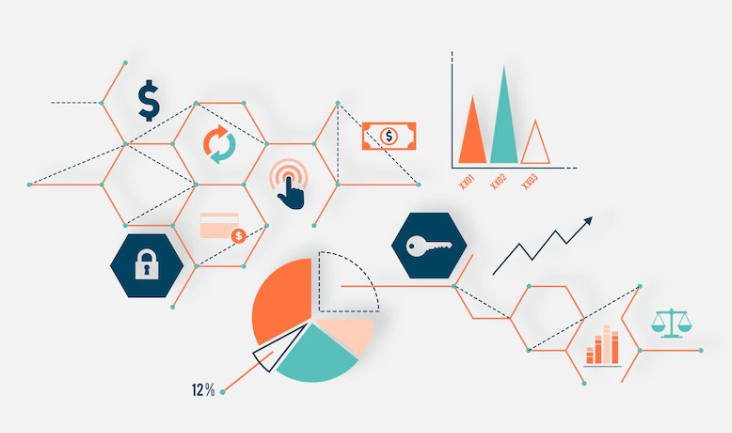
Big data analytics follows five steps to analyze any large datasets:
Data collection
This includes identifying data sources and collecting data from them. Data collection follows ETL or ELT processes.
ETL – Extract Transform Load
In ETL, the data generated is first transformed into a standard format and then loaded into storage.
ELT – Extract Load Transform
In ELT, the data is first loaded into storage and then transformed into the required format.
Data storage
Based on the complexity of data, data can be moved to storage such as cloud data warehouses or data lakes. Business intelligence tools can access it when needed.
A data warehouse is a database optimized to analyze relational data coming from transactional systems and business applications. The data structure and schema are defined in advance to optimize for fast searching and reporting. Data is cleaned, enriched, and transformed to act as the “single source of truth” that users can trust. Data examples include customer profiles and product information.
A data lake is different because it can store both structured and unstructured data without any further processing. The structure of the data or schema is not defined when data is captured; this means that you can store all of your data without careful design, which is particularly useful when the future use of the data is unknown. Data examples include social media content, IoT device data, and nonrelational data from mobile apps.
Organizations typically require both data lakes and data warehouses for data analytics. AWS Lake Formation and Amazon Redshift can take care of your data needs.
Data processing
When data is in place, it has to be converted and organized to obtain accurate results from analytical queries. Different data processing options exist to do this. The choice of approach depends on the computational and analytical resources available for data processing.
Centralized processing
All processing happens on a dedicated central server that hosts all the data.
Distributed processing
Data is distributed and stored on different servers.
Batch processing
Pieces of data accumulate over time and are processed in batches.
Real-time processing
Data is processed continually, with computational tasks finishing in seconds.
Data cleansing
Data cleansing involves scrubbing for any errors such as duplications, inconsistencies, redundancies, or wrong formats. It’s also used to filter out any unwanted data for analytics.
Data analysis
This is the step in which raw data is converted to actionable insights. The following are four types of data analytics:
1. Descriptive analytics
Data scientists analyze data to understand what happened or what is happening in the data environment. It is characterized by data visualization such as pie charts, bar charts, line graphs, tables, or generated narratives.
2. Diagnostic analytics
Diagnostic analytics is a deep-dive or detailed data analytics process to understand why something happened. It is characterized by techniques such as drill-down, data discovery, data mining, and correlations. In each of these techniques, multiple data operations and transformations are used for analyzing raw data.
3. Predictive analytics
Predictive analytics uses historical data to make accurate forecasts about future trends. It is characterized by techniques such as machine learning, forecasting, pattern matching, and predictive modeling. In each of these techniques, computers are trained to reverse engineer causality connections in the data.
4. Prescriptive analytics
Prescriptive analytics takes predictive data to the next level. It not only predicts what is likely to happen but also suggests an optimum response to that outcome. It can analyze the potential implications of different choices and recommend the best course of action. It is characterized by graph analysis, simulation, complex event processing, neural networks, and recommendation engines.
Impact of Data Lake & Analytics on AWS Services

AWS provides the broadest selection of analytics that fit all your data analytics needs and enables organizations of all sizes and industries to reinvent their business with data. From data movement, data storage, data lakes, big data analytics, log analytics, streaming analytics, business intelligence, and machine learning (ML) to anything in between, AWS offers purpose-built services that provide the best price-performance, scalability , and lowest cost.
Scalable Data Lakes
AWS-powered data lakes, supported by the unmatched availability of Amazon S3, can handle the scale, agility, and flexibility required to combine different data and analytics approaches. Build and store your data lakes on AWS to gain deeper insights than with traditional data silos and data warehouses allow.
Purpose-built for Performance and Cost Effective
AWS analytics services are purpose-built to help you quickly extract data insights using the most appropriate tool for the job, and are optimized to give you the best performance, scale, and cost for your needs.
Serverless and Easy to Use APIs
AWS has the most serverless options for data analytics in the cloud including options for data warehousing, big data analytics, real-time data, data integration, and more. We manage the underlying infrastructure so you can focus on solely on your application.
Unified Data Access, Security, and Governance
AWS lets you define and manage your security, governance, and auditing policies to satisfy industry and geography-specific regulations. With AWS, you can access your data wherever it lives, and we keep your data secure no matter where you store it.
Machine Learning (ML) Integration
AWS offers built-in ML integration as part of our purpose-built analytics services. You can build, train, and deploy ML models using familiar SQL commands, without any prior machine learning experience.
Top 10 AWS Data Analytics Services
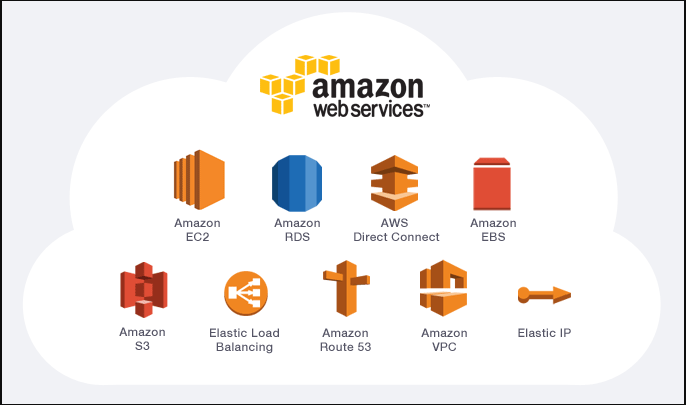
Before we start the discussion on most popular AWS platforms, it would be reasonable to go through some stats about them. It is interesting to note that AWS provides more than 70 services and the prices of the services have decreased considerably since the launch of AWS! The notable clients of AWS include Netflix, Reddit, NSA, and Expedia, which clearly show the demand for AWS.
Another noticeable factor that can be associated with the services of AWS is the addition of new features and services. Interestingly, AWS showed a 40% increase in new AWS features and services with the addition of 722 new services and features in one year. That seems to be quite a staggering growth, doesn’t it? Now, we shall move towards finding the top AWS services in demand!
The services provided by AWS are classified into several domains, and they include compute, database, messaging, migration, storage, management tools, network, and content delivery and security and identity compliance domains. However, it can be quite difficult to find out exactly which of these services are used widely.
Amazon Athena
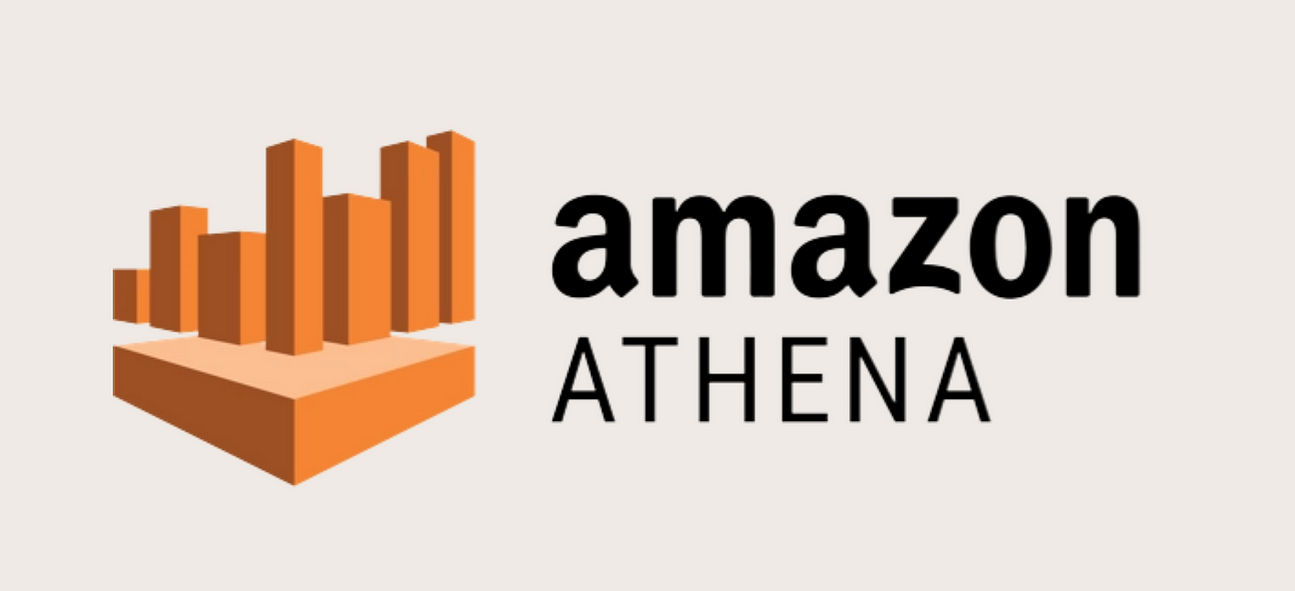
An interactive query service which helps in easy analysis of data stored in S3 with the help of SQL queries. It is server-less, hence having no infrastructure that needs to be managed. The user only pays for the queries they run.
Amazon EMR
It provides a Hadoop managed framework, which helps in quick and cost-effective method of processing large amounts of data across on-the-g- scalable EC2 instances. EMR notebooks are similar to Jupyter notebooks and provide an environment to develop and collaborate for dynamic querying and exploratory analysis if data. It is highly secure and offers reliability to handle a wide range of uses cases, which include, but are not limited to machine learning, scientific simulation, log analysis, web indexing, data transformations (ETL), and bioinformatics.
Amazon CloudSearch
It is a service managed by AWS Cloud, and it makes the process of setting-up, managing and scaling a search solution for a website or an application an easy task. It supports 34 languages and comes with features such as highlighting, auto-complete, and geospatial searchability.
Amazon ElasticSearch service
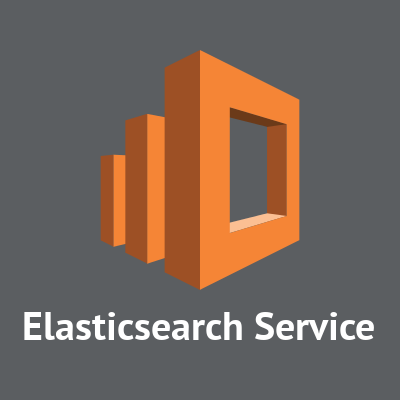
It helps ElasticSearch in the process of deploying, securing, operating and scaling data by searching, analyzing and visualizing this data in real-time. It comes with easy-to-use APIs which can be used for log analysis, full-text search, scaling requirements, monitoring applications, clickstream analytics, and security. It can also be integrated with open-source tools such as Kibana, Logstash which help in ingesting data and visualization of data respectively.
Amazon Kinesis
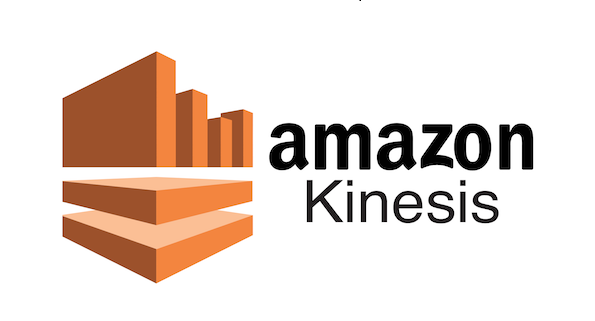
It makes the process of collecting, processing, and analyzing real-time Kinesis data analytics an easy task. This helps in achieving real-time insights to data so that this data can be analyzed, and actions can be taken based on the insights quickly. Real-time data including video, audio, application logs, website clickstreams, IoT telemetry data (meant for machine learning) can be ingested, and this data can be responded to immediately in contrast to waiting for all the data to arrive before beginning pre-processing on it. It currently offers services like- Kinesis Data Firehose, Kinesis Data Analytics, Kinesis Data Streams, and Kinesis Video Streams.
Amazon redshift
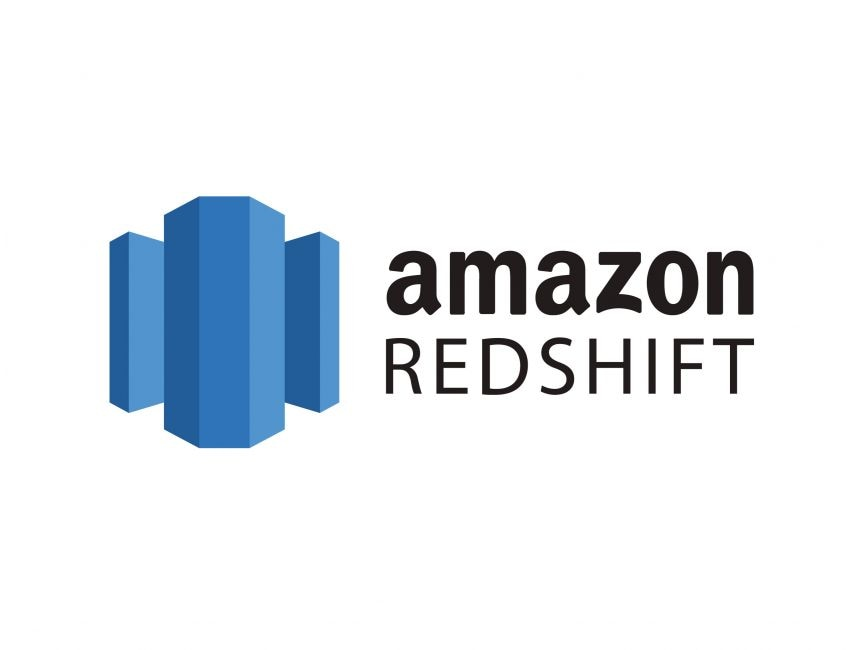
It is a quick, and scalable data warehouse which helps in analyzing user data in a cost-efficient and simple manner. It delivers 10 times quicker performance in comparison to other data warehousing services, since it uses machine learning, massively parallel query execution and columnar storage on high-performance disks. Petabytes of data can be queried upon, and a new data warehouse can be setup and deployed in a matter of minutes.
Amazon QuickSight
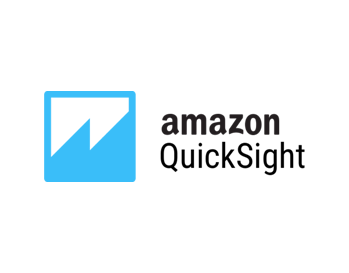
It is a cloud powered business intelligence service, which is fast, and helps in delivery insights to people in an organization. It allows users to create and publish interactive visual dashboards which can be accessed via mobile devices and browsers. These visual dashboards can be integrated with other applications thereby providing customers with powerful self-serving real time data analysis service.
AWS data pipeline
It helps process and move data between different AWS resources (compute and storage devices). Data can be regularly accessed from the place it is stored, it can be transformed and processed at scale. The result of this data processing can be transferred to other AWS services such as S3, RDS, DynamoDB, and EMR. It helps in the creation of complex data processing workloads which provide facilities such as high fault tolerance, high availability and repeatability.
AWS Glue
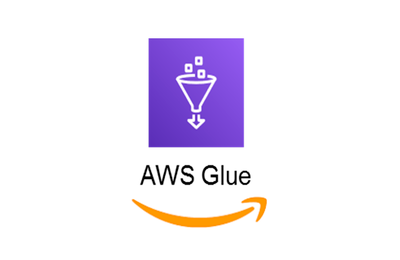
It is a completely managed ETL service (Extract, Transform, Load) which helps users prepare and load their data for the purpose of analysis. An ETL job can be set up and run with a few mouse clicks from the AWS Management Console itself. Glue can be pointed to the location of data stored, and it discovers the data and its metadata and stores it in Glue Catalog. Once the data is in the catalog, it can be searched, queried, and made available for ETL process.
Amazon Lake Formation
It is a service that helps in securing data lake. Data Lake can be visualized as a centralized, customized and secured data repository which stores this data in the original form as well as a processed form meant for data analysis. It helps combine various types of analytics that help in gaining deeper insights to data thereby helping make better business decisions. But the process of setting up and managing a data lake requires a lot of manual efforts. But Lake Formation makes this process an easy one by collecting data and cataloguing it. This data is then classified using ML algorithms as well as providing security for sensitive data.
Amazon Managed streaming for Kafka (MSK)
It is a service that helps in building and running applications that use Apache Kafka. It is fully managed and helps process streaming data. Apache Kafka is open-source and is used to build real-time streaming data pipelines and application. With the help of MSK, Kafka API can be used to populate data lakes, reflect changes in the database and use machine learning to power other applications.