As per Gartner's prediction back in 2018, around 85% of data science-based ventures will collapse till 2022. Moreover, the Economist forecasted in their study that although 70% of business leaders have stated analytics as extremely high quality data or valuable resource, only 2% remarked it for achieving broad positive results.
The three primary reasons differentiating the usage of important data for future use between expectations and facts are:
Lack of definition for the problem
Inability of reaching to a solution from different perspectives
Unable to transform raw data into actionable insights for changing complete view of business executives.
If you want to accomplish success in big data or implement effective data management then it is essential to track and maintain the system from real time data sources, data collection, and data accuracy, to data storage, data protection and data analysis.
In this blog, we'll be sharing some of the best practices for implementing data management system using different tools to improve customer experience and achieve your business goals.
How Effective is Data Management?
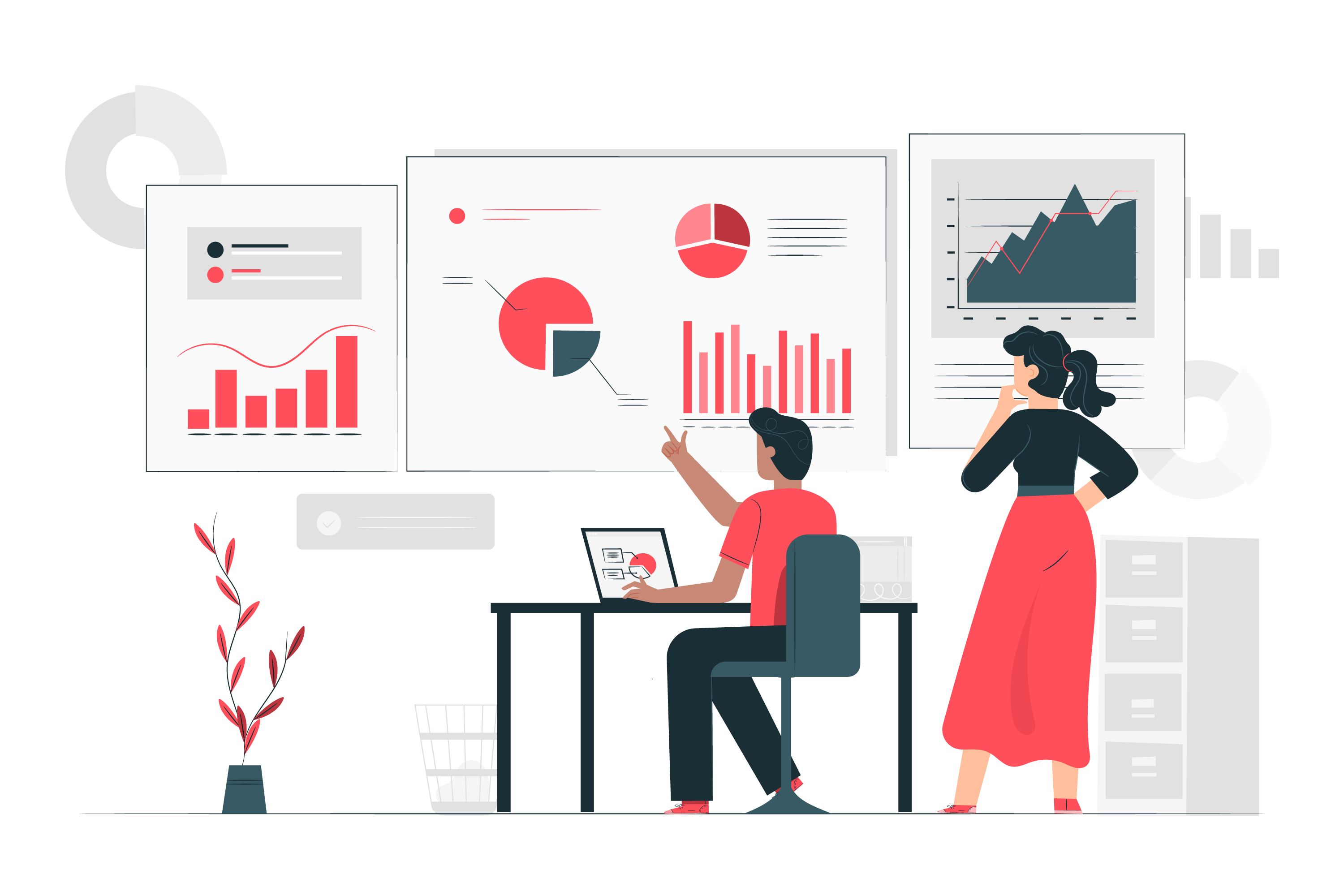
Before jumping on to the data management best practices, you must know how data management has impacted the productivity, efficiency, and data security in many organizations.
Data management is the detailed process of data governance framework that deals with data storage, data security, and ensure data quality which can help your business to make well-informed and sustained decisions. As the main goal of most data managers is to boost your business analytics, and most organizations consume data at effective data management can also help you achieve that since you can have complete data access which can be utilized for your benefits.
Nowadays, some of the best data management software makes sure that the data set you get is reliable and updated to drive successful decisions. These data management software assists with data preparation, cataloging, search, governance, and other tasks so that individuals may easily locate the data they require for analysis.
Key Components of Data Management
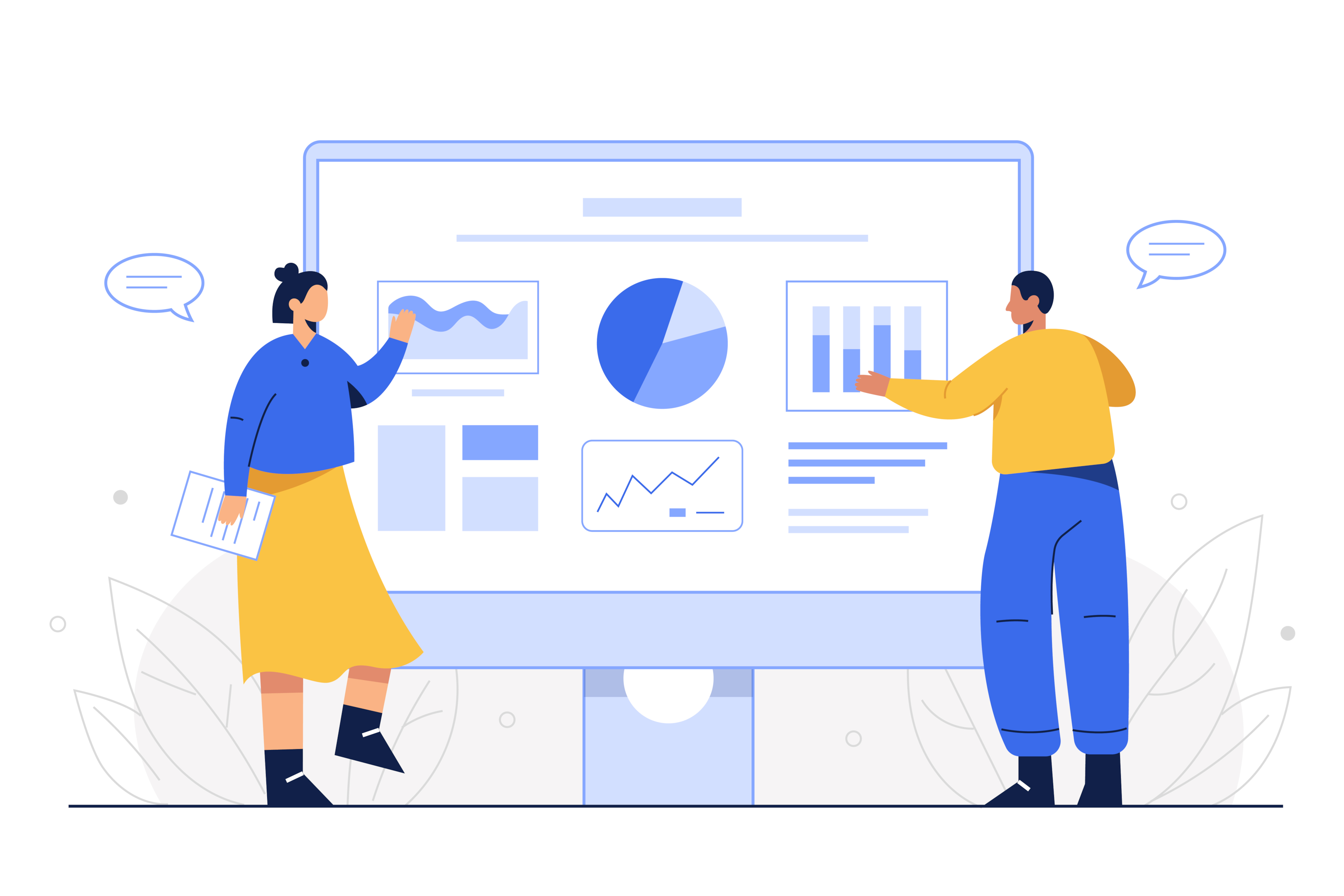
For effective data governance, you must know all the data management elements that not only assist in managing data but also look for different data sources for ensuring complete usage of your business data set:
Data Modeling
It depicts how data flows across systems and the relationships between data items.
Data Integration
It combines information from several sources for use in operations and analysis.
Data Stewardship
Data stewardship establishes policies and procedures to guarantee that data is uniform across an organization.
Data Quality management
This employs quality data management software which aims to correcting data mistakes and discrepancies.
Master Data management
MDM or master data management creates a standard set of reference information on factors like clients and products.
Most data governance teams integrate high-end data management software to utilize all the above-defined key components within their data management strategy across different departments.
Top 8 Best Practices to Improve Data Management Processes
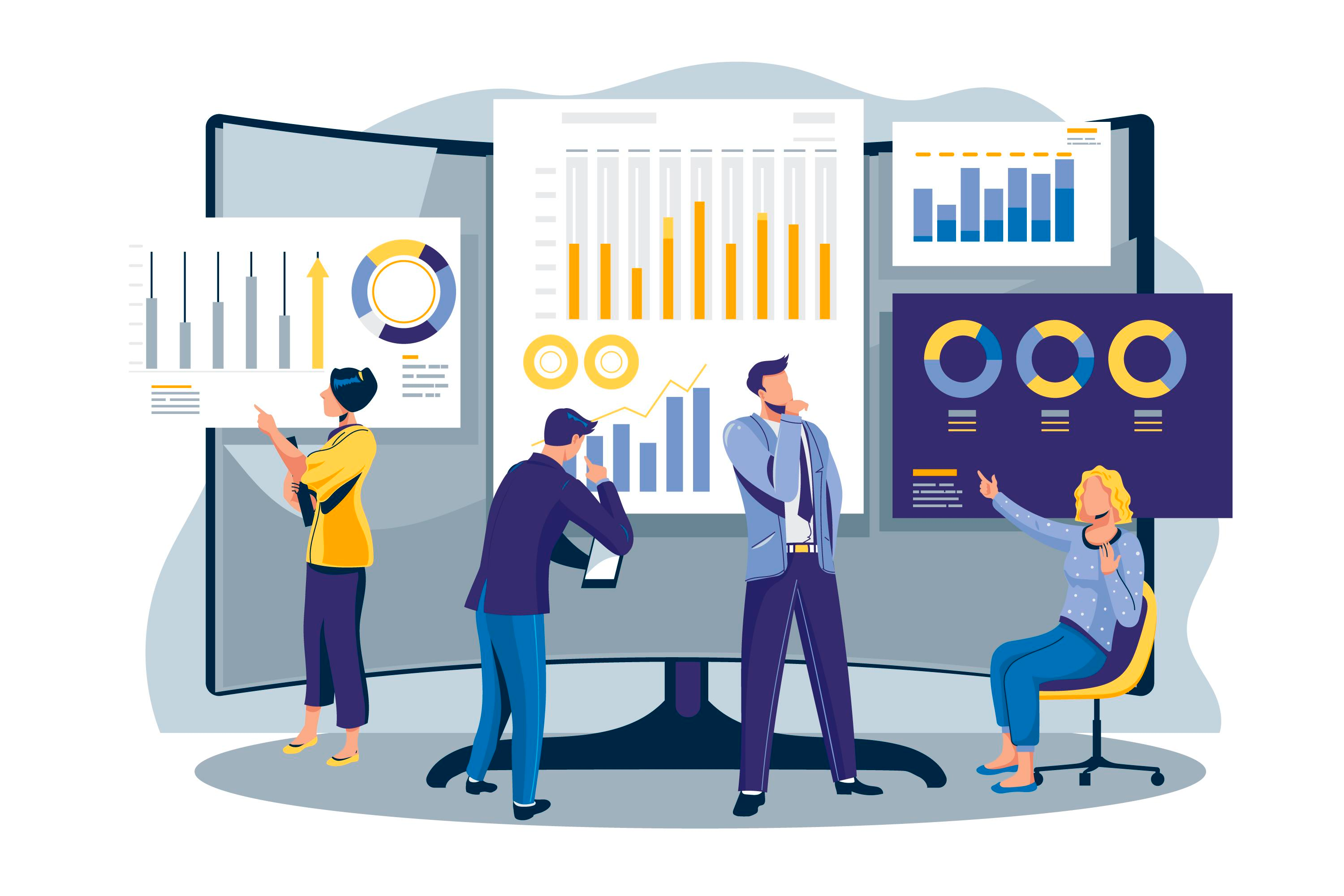
Following are some of the data management best practices implemented by data managers to boost growth driven decisions in this constantly changing market.
Analyze How Data Quality Impacts Your Decisions
Determine how business processes, key performance indicators (KPIs), and data assets are clearly related. Create a list of the data quality problems the organization is now experiencing and how they affect sales and other business KPIs.
Data and analytics executives can start developing a targeted data quality management plan that precisely defines the scope, the list of stakeholders, and a high-level investment plan once they have established a clear connection between data as an asset and the improvement requirements.
Define Standards to Focus on Data Quality
First and foremost, it's critical to comprehend what "best fits" the organization in order to increase data quality. The business is in charge of defining what is considered to be "good." Leaders in data and analytics (D&A) must regularly consult with business stakeholders to understand their expectations.
There may be differences in standards and expectations for the data management plan across different business lines using the same data, such as customer master data.
Prioritize Data Security
Consumers whose information has been compromised may require credit monitoring from businesses that experience a data breach. Remedial actions must be taken, including paying for new cards, repairing identity theft, and meeting additional compliance standards. As a result, it is obvious how important data privacy is to improve data quality.
The safeguarding measures put in place to protect databases, data sets, websites, and computers from unauthorized access are referred to as data security measures. It is important to prioritize data protection against data loss or corruption to improve your consumer experience.
Reduce Duplicate Data
The growth of your organization is the responsible for duplicate data. Most managers would often prioritize investing in accounting software as it expands. A customer relationship management (CRM) package, distribution software, project management software, HR software, and several other ad hoc platforms and software solutions are typically used after this.
These systems frequently come from several suppliers and hardly ever communicate with one another. Because of these disjointed systems, each department functions independently, entering its own duplicate records into its own software. As you may expect, a lot of this data is redundant.
Use Data Profiling Often
When it comes best practices, data profiling is a must. Data quality profiling is the process of looking at data from a source that already exists and compiling facts about the data. It facilitates the identification of necessary corrective measures and offers insightful data that can be shared with the organization to inspire the development of improvement strategies.
Data profiling can be useful in determining which data quality problems need to be rectified right away and which can wait. Nonetheless, it is a continuous process. Depending on the availability of resources, data problems, etc., data profiling should be performed as regularly as possible.
Profiling, for instance, can show that important client contact details are lacking. A high number of customer complaints may have been directly caused by this missing information, which would make providing good customer service challenging.
Carefully Consider Metadata for Data Sets
Cleaning, categorizing, and organizing unstructured data for accuracy, integrity, consistency, and usefulness is known as metadata management.
It gives you the ability to trust your metadata and is the key to categorizing the data assets in your company. You can create a metadata repository and allow metadata discovery, lineage mapping, and governance using tools for managing metadata.
To begin managing metadata, you must create a metadata strategy that makes use of all available types of metadata and establish a setting that makes it simple to find, comprehend, and use metadata.
Create a Data Recovery Strategy
Anybody who works on the Information Technology for their firm should be aware by this point that unanticipated mishaps, emergencies, and disasters can strike a business when it least expects it.
Sadly, such occurrences may result in the loss of important data. Data recovery is frequently essential for corporate operations and is frequently regarded as the most crucial asset to concentrate on in a larger disaster recovery plan.
The process to access lost, damaged, or otherwise inaccessible data from a network device such as a computer, smartphone, storage device, or server, and putting it back in its original position is known as data recovery.
Getting Started with Data Management Software
Data from many data sources are combined and managed by data management software. Once the data has been recovered, cleaned, converted, and integrated, you can access it naturally without compromising its integrity.
Important business objectives, such as data integration, extraction, warehousing, virtualization, etc., are accomplished utilizing reliable ETL (extract, transform, and load) solutions. When repurposing data to meet business goals, the software's ability to execute several queries is crucial.
The management of software data and information falls outside the purview of the IT staff. It's a business decision that will help and support your company in all of its undertakings. It's essential to use these technologies to make the most of the information your business has to offer. All users have secure ways to access and use data whenever they want, which lowers human error.
Data modelling, data integration, and multimedia data are made possible by a variety of database management systems with various architectures and application specializations.